If computers could become ‘smart’ enough to recognize who is talking, that could allow them to produce real-time transcripts of meetings, courtroom proceedings, debates, and other important events. In the dissertation that will allow him to receive his Ph.D. at Commencement this year, Brian Reggiannini found a way to advance the state of the art for voice- and speaker-recognition.
Everyone does signal processing every day, even if we don’t call it that. With friends at a sports bar, we peer up at the TV to see the score, we turn our head toward the crashing sound when a waitress drops a glass, and perhaps most remarkably, we can track the fast-paced banter of all the people in our booth, even if we’ve never met some of the friends-of-friends who have insinuated themselves into the scene.
Very few of us, however, could ever get a computer to do anything like that. That’s why doing it well has earned Brian Reggiannini a Ph.D. at Brown and a career in the industry.
In his dissertation, Reggiannini managed to raise the bar for how well a computer connected to a roomful of microphones can keep track of who among a small group of speakers is talking. Further refined and combined with speech recognition, such a system could lead to instantaneous transcriptions of meetings, courtroom proceedings, or debates among, say, several rude political candidates who are prone to interrupt. It could help the deaf follow conversations in real-time.
If only it weren’t so hard to do.
But Reggiannini, who came to Brown as an undergraduate in 2003 and began building microphone arrays in the lab of Harvey Silverman, professor of engineering, in his junior year, was determined to advance the state of the art.
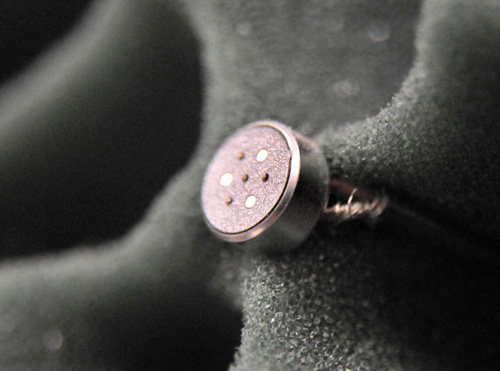
Real-time tracking of who’s talking. With the right algorithms and signal processing software, an array of button-size microphones placed around the perimeter of a room can identify, follow, and record each of several people as they move about, interrupt each other, and converse. Image credit: Frank Mullin/Brown University
The specific challenge he set for himself was real-time tracking of who’s talking among at least a few people who are free to rove around a room. Hardware was not the issue. The test room on campus has 448 microphones all around the walls and he only used 96. That was enough to gather the kind of information that allows systems – think of your two ears – to locate the source of a sound.
The real rub was in devising the algorithms and, more abstractly, in realizing where his reasoning about the problem had to abandon the conventional wisdom.
Previous engineers who had tried something like this were on the right track. After all, there is only so much data available in situations like this. Some tried analyzing accents, pronunciation, word use, and cadence, but those are complex to track and require a lot of data. The simpler features are pitch, volume, and spectral statistics (a breakdown of a voice’s component waves and frequencies) of each speaker’s voice. Systems can also ascertain where a voice came from within the room.
Snippets, not speakers
But many attempts to build speaker identification systems (like the voice recognition in your personal computer) have relied on the idea that a computer could be extensively trained in “clean,” quiet conditions to learn a speaker’s voice in advance.
One of Reggiannini’s key insights was that just like a politician couldn’t possibly be primed to recognize every voter at a rally, it’s unrealistic to train a speaker-recognition system with the voice of everyone who could conceivably walk into a room.
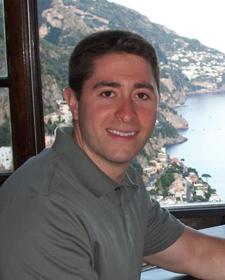
Brian Reggiannini. “We’re trying to teach a computer how to do something that we as humans do so naturally that we don’t even understand how we do it.” Image credit: Brown University
Instead, Reggiannini sought to build a system that could learn to distinguish the voices of anyone within a session. It analyzes each new segment of speech and also notes the distinct physical position of individuals within the room. The system compares each new segment, or snippet, of what it hears to previous snippets. It then determines a statistical likelihood that the new snippet would have come from a speaker it has already identified as unique.
“Instead of modeling talkers, I’m going to instead model pairs of speech segments,” Reggiannini recalled.
A key characteristic of Reggiannini’s system is that it can work with very short snippets of speech. It doesn’t need full sentences to work at least somewhat well. That’s important because it’s realistic. People don’t speak in florid monologues. They speak in fractured conversations. No way! Yes, really.
People also are known to move around. For that reason position as inferred by the array of microphones can be only an intermittent asset. At any single moment in time, especially at the beginning of a session, position helpfully distinguishes each talker from every other (no two people can be in the same place at the same time), but when people stop talking and start walking, the system necessarily loses track of them until they speak again.
Reggiannini tested his system every step of the way. His experiments included just pitch analysis, just spectral analysis, a combination of the two, position alone, and a combination of the full speech analysis and position tracking. He subjected the system to a multitude of voices, sometimes male-only, sometimes female-only, and sometimes mixed. In every case, at least until the speech snippets became quite long, his system was better able to discriminate among talkers than two other standard approaches.
That said, the system sometimes is uncertain and in cases like that it defers assigning speech to a talker until it is more certain. Once it is, it goes back and labels the snippets accordingly.
It’s no surprise that the system would err, or hedge, here and there. Reggiannini’s test room was noisy. While some systems are fed very clean audio, the only major concessions that Reggiannini allowed himself were that speakers wouldn’t run or jump across the room and that only one would speak from the script at a time. The ability to filter individual voices out from within overlapping speech is perhaps the biggest remaining barrier between the system remaining a research project and becoming a commercial success.
A career in the field
While the ultimate fate of Reggiannini’s innovations is not yet clear, what is certain is that he has been able to embark on a career in the field he loves. Since leaving Brown last summer he’s been working as a digital signal processing engineer at Analog Devices in Norwood, Mass., which happens to be his hometown.
Reggiannini has yet to work on an audio project, but that’s fine with him. His interest is the signal processing, not sound per se. Instead he’s applied his expertise to challenges of heart monitoring and wireless communications.
“I’ve been jumping around applications but all the fundamental signal processing theory applies no matter what the signal is,” he said. “My background lets me work on a wide range of problems.”
After seven years and three degrees at Brown, Reggiannini was prepared to pursue his passion.
– By David Orenstein
*Source: Brown University