UCLA, Purdue computer scientists develop technique for combining massive sets of research data
As the field of “big data” has emerged as a tool for solving all sorts of scientific and societal questions, one of the main challenges that remains is whether, and how, multiple sets of data from various sources could be combined to determine cause-and-effect relationships in new and untested situations. Now, computer scientists from UCLA and Purdue University have devised a theoretical solution to that problem.
Their research, which was published in the Proceedings of the National Academy of Sciences, could help improve scientists’ ability to understand health care, economics, the environment and other areas of study, and to glean much more pertinent insight from data.
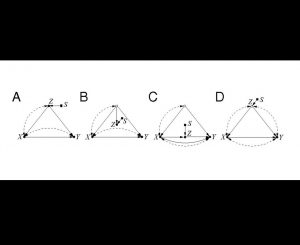
Data fusion. Bareinboim and Pearl discovered how to estimate the effect of one variable on another when data come from disparate sources that differ in yet another variable, Z. Image credit: Judea Pearl and Elias Bareinboim (Click image to enlarge)
The study’s authors are Judea Pearl, a distinguished professor of computer science at the UCLA Henry Samueli School of Engineering and Applied Science, and Elias Bareinboim, an assistant professor of computer science at Purdue University who earned his doctorate at UCLA.
Big data involves using mountains and mountains of information to uncover trends and patterns. But when multiple sets of big data are combined, particularly when they come from studies of diverse environments or are collected under different sets of conditions, problems can arise because certain aspects of the data won’t match up. (The challenge, Pearl explained, is like putting together a jigsaw puzzle using pieces that were produced by different manufacturers.)
For example, researchers might be interested to combine data about people’s health habits from several unrelated studies — say, a survey of Texas residents; an experiment involving young adults in Kenya; and research focusing on the homeless in the Northeast U.S. If the researchers wanted to use the combined data to answer a specific question — for example, “How does soft drink consumption affect obesity rates in Los Angeles?” — a common approach today would be to use statistical techniques that average out differences among the various sets of information.
The new study claims that these statistical methods blur distinctions in the data, rather than exploiting them for more insightful analyses.
“It’s like testing apples and oranges to guess the properties of bananas,” said Pearl, a pioneer in the field of artificial intelligence and a recipient of the Turing Award, the highest honor in computing. “How can someone apply insights from multiple sets of data, to figure out cause-and-effect relationships in a completely new situation?”
To address this, Bareinboim and Pearl developed a mathematical tool called a structural causal model, which essentially decides how information from one source should be combined with data from other sources. This enables researchers to establish properties of yet another source — for example, the population of another state. Structural causal models diagram similarities and differences between the sources and process them using a new mathematical tool called causal calculus.
The analysis also had another important result — deciding whether the findings from a given study can be generalized to apply to other situations, a century-old problem called external validity.
For example, medical researchers might conduct a clinical trial involving a distinct group of people, say, college students. The method devised by Bareinboim and Pearl will allow them to predict what would happen if the treatment they were testing were given to an intended population of people in the real world.
“A problem that every scientist in every field faces is having observations from surveys, laboratory experiments, randomized trials, field studies and more, but not knowing whether we can learn from those observations about cause-and-effect relationships in the real world,” Pearl said. “With structural causal models, they can ask first if it’s possible, and then, if that’s true, how.”
*Source: University of California